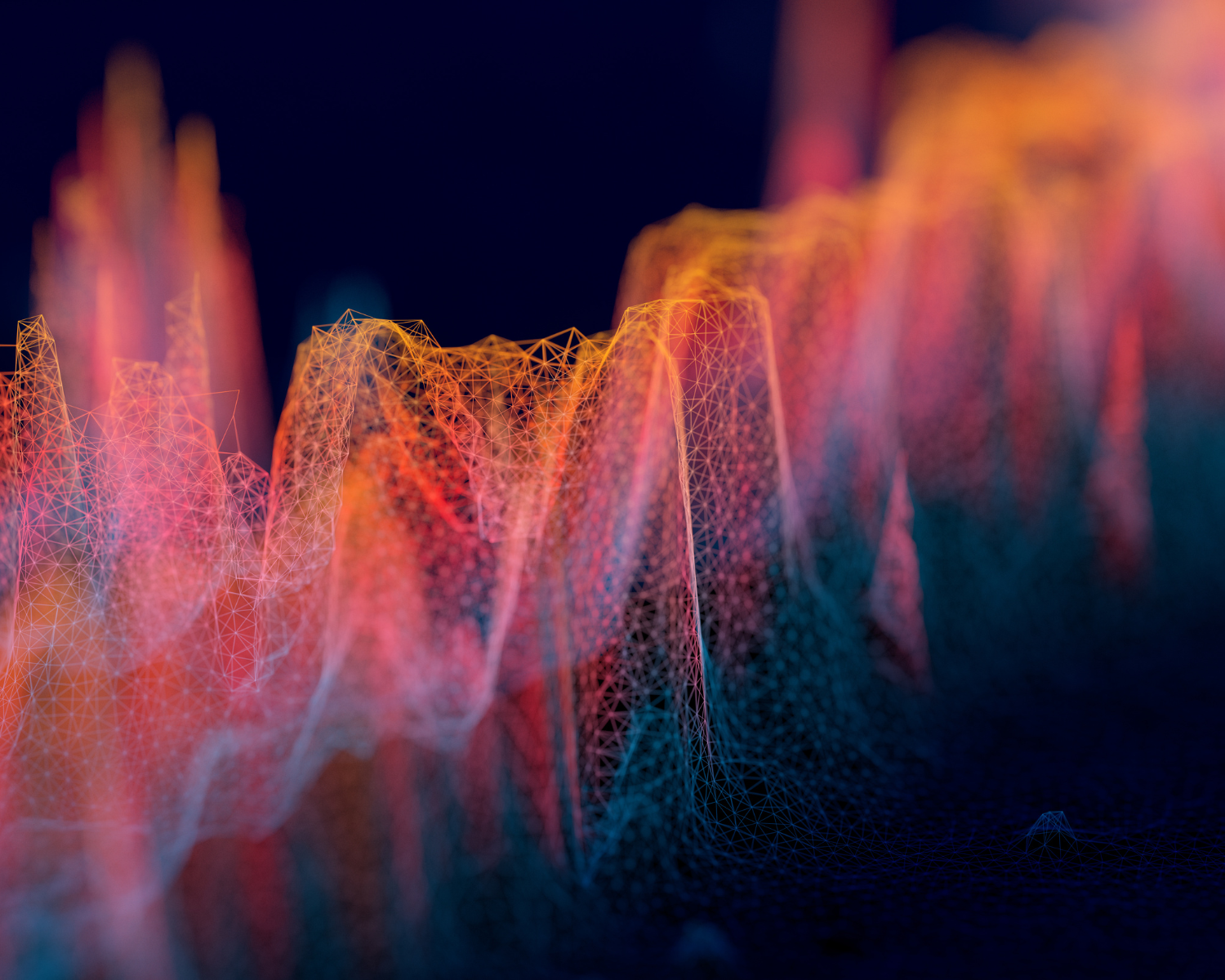
Rachel Augustin, Senior Consultant, Presents Research & Analysis at the American Public Health Association’s Annual Meeting in Boston.
The American Public Health Association (APHA) will see Rachel’s presentation, which focuses on research she has done to show how data science informs suicide prevention efforts. During her time at the meeting, she will specifically describe the analytical steps and conclusions behind her abstract submission, “Behavioral Healthcare Use Prior to Suicide in Philadelphia’s Medicaid Population.”
Because the analysis aimed to describe behavioral healthcare service use before suicide, Rachel used the following data science methods: 1) data mining, scoping large datasets to identify and extract data, and 2) data linkage, to connect two or more independent datasets. Using these methods, she conducted a retrospective analysis of deaths recorded as suicide between 2003 and 2018 by the Philadelphia Medical Examiner’s Office (MEO). She matched the data to Medicaid behavioral health claims and eligibility data during the same timeframe. An essential part of this entity resolution (i.e., matching) process was to acquire a linear timeline of behavioral health care use in the 7-, 30-, 90, and 365- days leading up to suicide (the index date) for each suicide decedent.
Statistical Analyses Examined
- The association between the category of behavioral healthcare service and rate of contact preceding suicide.
- Demographic characteristics on use of behavioral healthcare services.
- Intersections of race/ethnicity (Black, Hispanic/Latino, and White) and gender with age on treatment use.
All analyses were conducted in SQL using SAS 9.
Leveraging Data and Insights
Like other studies, the results showed that outpatient mental health is a critical contact point. Any intervention strategy will need to engage older adult men, found unlikely to engage with the mental health system.
Rachel’s research aligns with her interests in leveraging data to glean insights and improve the quality and experience of care. She has a background in public health with expertise in analyzing large-scale data using statistical and machine-learning methodologies.
Of note, her research, “A Behavioral Health Population Pyramid: Using Cluster Analysis,” as part of her doctoral studies at the Drexel University Dornsife School of Public Health, presented at the 2019 APHA conference, used mini-batch K-means cluster analysis to categorize behavioral health service users to inform care management strategies at a Behavioral Health Medicaid Managed Care Organization. It was selected as a solution because it was ideal for finding similar attributes among a diverse group of over 700,000 Medicaid beneficiaries.
K-means cluster analysis is an unsupervised learning algorithm that groups observations into a set number of clusters or groups (denoted by k, k=5, represents 5 clusters, and so forth). Briefly, clustering occurred as follows:
- Data sourced from Medicaid behavioral health claims.
- Data pre-processing involved transforming variables needed for model input.
-
- Service mapping: categorizing individual-level services into broader service use categories (e.g., hospital stay mapped to the acute services category). Service categories acted as the input variables for clustering.
- The intensity of behavioral health service use (total time spent by each Medicaid beneficiary in a service category) defined the distance between and within clusters.
- The data set was standardized.
- Used the robust Mahalanobis distance outlier detection to reduce potential model-fit errors.
- Used principal component analysis (PCA) to reduce the dimensionality of highly correlated variables.
- K-means clustering occurred on the training data set, and the resulting model was evaluated using the test data set. Note: For computational performance, mini-batch K-means was used because it does not iterate over the entire dataset.
- Post-hoc analyses were conducted using demographics and diagnoses to assess the resulting clusters.
Results of the Analysis
The results of the analysis revealed distinct service use profiles. For example, most Medicaid beneficiaries used one service category compared to multiple service users. Regarding future steps, she hopes to continue her work on the cluster analysis and build it into a decision-support system that will allow health providers to make real-time decisions on behavioral healthcare interventions.
Rachel brings a unique perspective to The Carnrite Group. Her experience making sense of high-volume historical and real-time data and the approaches needed cuts across all industries. Rachel plans to leverage her expertise to support similar issues facing clients of The Carnrite Group.